FDA issues initial draft guidance on leveraging clinical and billing real-world data sources for regulatory use
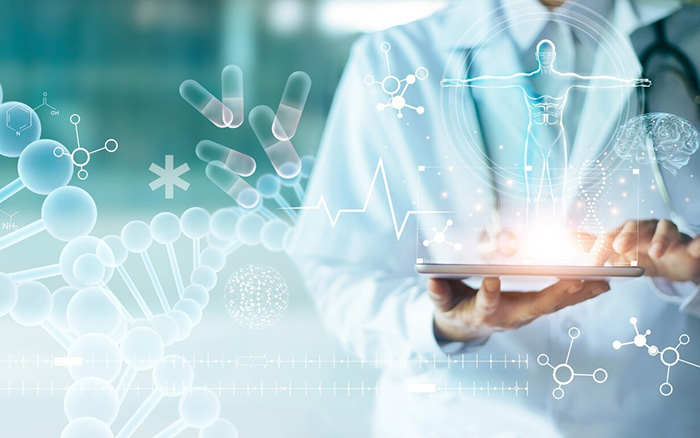
Drug developers seeking to leverage data from electronic health records or medical claims for regulatory use need to be careful about the relevance and reliability of the baseline data itself, explains the FDA in a new draft guidance in which it offers its initial thinking about how it considers – and may, in the future, assess – the quality of real-world data sources.
Executive IQ Brief
- How Things Work Now: Under the Federal Food, Drug, and Cosmetic (FD&C) Act, approval of a drug or biologic by the FDA requires that the product demonstrate “substantial evidence” that it is safe and effective for use. While this has traditionally meant two well-controlled clinical trials, both industry and the FDA have been exploring the use of real world data (RWD) – or data collected from routine interactions with the health care system – and evidence generated by analyses of this data (RWE) to support life sciences regulation.
- What’s New: The FDA has now issued draft guidance for drug developers (the guidance is co-sponsored by CBER, CDER and OCE) on the specific considerations for leveraging data from electronic health records (EHRs) and medical claims into RWD. While the guidance does not provide recommendations on the conversion of RWD into RWE, it delineates recommendations – and areas of concern – for transitioning source data into RWD for potential regulatory use.
- Impact: It’s important to understand what the draft guidance is – and what it’s not. This particular document outlines key considerations that a developer or researcher should keep in mind when parsing through data from EHR/claims sources and proposing any sort of analyses on the data – in order to “maximize completeness and accuracy” of the data itself – but doesn’t include concrete recommendations on the analyses. Rather, the guidance document focuses on the baseline data itself, setting expectations and a preliminary rubric by which the agency may consider the quality and sufficiency of underlying RWD datasets when analyses of these data are used in regulatory submissions. The guidance is very likely a first step, outlining a series of considerations for curating datasets from RWD sources rather than specific recommendations for the analyses of RWE. However, with new commitments under PDUFA VII and other agency initiatives related to RWD validation are likely to inform, and expedite, the path ahead
What’s Next: The guidance is open for comment until November 29.
- FDA: Real-World Data: Assessing Electronic Health Records and Medical Claims Data To Support Regulatory Decision-Making for Drug and Biological Products
- Docket No. FDA-2020-D-2307
- Guidance Document Backup Download: 34193775fnl.pdf
Regulatory Background
Under the Federal Food, Drug, and Cosmetic (FD&C) Act, approval of a drug or biologic by the FDA requires that the product demonstrate “substantial evidence” that it is safe and effective for use. The FDA has traditionally interpreted the term “substantial evidence” as two well-controlled clinical trials, although it often allows a single trial to provide “substantial” evidence when multiple trials would be infeasible (such as for rare diseases with small populations or hard-to-treat and quickly debilitating diseases).
[AgencyIQ subscribers can read analysis of the changing evidence standards here.]
While randomized, controlled clinical trials (RCTs) remain the gold standard for the generation of evidence, there are other forms of evidence that also inform regulatory decision making. This “real world data” (RWD) can be collected and analyzed to generate “real world evidence” (RWE).
FDA defines RWD as “the data relating to patient health status and/or the delivery of health care routinely collected from a variety of sources,” including insurance and medical claims, electronic health or medical records, and disease or clinical registries. It defines RWE as “the clinical evidence regarding the usage and potential benefits or risks of a medical product derived from analysis of RWD.”
The basic idea of using RWE is simple: Once approved, many products are used both in accordance with their label and “off-label.” Some products may be more useful than initially thought, while others may have other uses that may not have been studied. Data about these uses could help a company to expand their approved label without needing to conduct extensive clinical testing.
A key source of potential RWE is data gathered during the course of medical treatment through an Electronic Health Record (EHR) or Electronic Medical Record (EMR). However, the validation and collection of that data represents significant operational and analytical hurdles.
As noted by many FDA senior staffers in a 2016 piece published in the New England Journal of Medicine, one of the most critical challenges is data validation. A database with poor-quality data (i.e., the inputs) likely will lead to poor-quality conclusions (i.e., the outputs). Studies should also incorporate longstanding evidentiary concepts, such as randomization, to minimize intentional or unintentional bias.
However, the control of some elements is inherently difficult. RCTs are well-controlled and closely regulated, with specific protocols for everything from the researcher’s language when surveying patients to clinical researcher’s clothing around samples. The intent is to minimize the number of external “confounding” factors that might influence the outcomes of the study other than the treatment itself. However, these types of factors are not generally controllable outside of the trial environment.
EHRs and medical claims data, in contrast, is almost never as robust as data obtained from a clinical trial and typically reflect an extraordinary amount of confounding factors. The people inputting the data may not have been trained in a standardized way. Different doctors may disagree about how to diagnose a particular condition. Certain inputs may have been done in error or out of expediency. Some procedures may be “up-coded” for reimbursement purposes. Medical records rarely track every single medical interaction that a person has with the health care system, and a patient may have unreported experienced not captured in their health record or submitted for reimbursement to their health insurer.
Even still, stakeholders – including Congress – have been pushing the FDA to be more willing to accept RWE as part of regulatory submissions. Under the 21st Century Cures Act of 2016, the FDA was directed to consider how RWD and RWE could be more broadly applied for regulatory use. However, progress in leveraging these data has been slow for the drug industry.
The agency currently maintains limited guidance for drug developers on the use of RWE. In addition to a guidance document that outlines how to denote that a certain application includes RWE, there are two applicable guidance documents for drug sponsors.
The first was finalized in 2013 and outlines best practices for conducting and reporting pharmacoepidemiologic safety studies using electronic health care data. This guidance included recommendations on how to leverage data from electronic medical records and administrative claims data to conduct these particular studies, which are typically observational studies that assess risks from drug exposures.
In addition, in 2018 the agency finalized a guidance document on the use of electronic health record data for clinical investigations. This guidance provides a high-level description of EHR systems and their governance, practices and use. An enterprise-wide guidance, it outlines key terms related to EHR systems, considerations for validating data captured in EHRs and lays out a series ‘of “best practices” for these data.
Regulatory Context
Over the past several years – and particularly accelerated during the pandemic – the drug industry has expressed significant interest in using RWE for regulatory purposes. The general hope is that RWE could be used to augment, accelerate or even replace the need to run certain studies or trials, including both prior to and following approval.
However, while the use of RWE is generally well established for the medical device industry, the drug industry has had limited opportunities to leverage this type of evidence.
In recent years, increasingly advanced data systems and larger volumes of data have paved the way for the wider use of RWE in drug research, especially as additional stakeholders are engaged in building a comprehensive health care data system in the U.S. For example, the FDA’s Sentinel Initiative, a real-world data post-market surveillance system that operates as a distributed data network, has served as a forum for pressure-testing certain real-world analytical methods, bringing in new data and working to increase regulatory understanding of certain real-world data sets (including EHR data).
There are other signs that the FDA’s adoption of RWE is set to increase substantially in the coming years. The recent commitment letter for the next iteration of the drug user fee program (PDUFA VII) includes a planned pilot program, targeted for implementation by the end of 2022, under which the FDA would focus on the development of agency processes to promote consistency related to RWE decision-making and promote “awareness of characteristics of RWE that can support regulatory decisions by allowing FDA to discuss study designs considered in the Advancing RWE Program in a public forum.” Under the program, the agency would solicit proposals for RWE-based research programs from sponsors twice a year, which would be reviewed and discussed in a public forum. Effectively, the pilot is intended to set a foundation for the rubric by which the FDA will assess RWE research programs for regulatory use.
In addition, FDA is also set to expand upon the use of RWE to meet post-approval study commitments, known as Post-Marketing Requirements (PMRs), under the PDUFA VII agreement.
What’s New
This week the FDA issued a draft guidance that outlines recommendations for sponsors that are considering leveraging data from two specific sources of real-world data: EHRs and medical claims. In accordance with the FDA’s RWE Program, the guidance applies to RWD-based research processes for both expanded labeling (of already approved products) and use of these analyses to support post-market activities – not new approvals for novel products.
Overall, the guidance outlines considerations for the selection, curation and quality assurance practices that a sponsor would need to think about when proposing to leverage RWD from EHRs or claims for the purposes of an RWE analyses. However, it doesn’t include recommendations about choosing certain study designs or how to appropriately conduct statistical analyses of RWD (i.e., to turn RWD into RWE) – instead deferring to the FDA’s intended future guidance on this subject. The agency states that the new draft is intended to “complement” the 2013 guidance “by expanding certain aspects of that guidance related to the selection of data sources,” while outlining additional considerations for using EHR and claims data in clinical studies (notably, the 2013 guidance relates solely to using EHR data for safety studies).
More broadly, the new draft also provides an “overview of considerations” about the use of data from these sources “more generally.” For example, the agency notes that any sponsor considering leveraging data should “see[k] FDA input before conducting the study” and predefine all “essential elements” of the study’s design, analytical plan, conduct and reporting – as well as how the RWD source was used to ascertain each data element outlined in the final study report.
In addition, the guidance includes sections on the data sources themselves, provides recommendations on selecting information and transitioning it into RWD through defined processes, the specific elements for consideration in baseline study designs and the ensuring the data quality, in order to “maximize the completeness and accuracy of the data derived from EHRs and medical claims for clinical studies.”
What are the challenges of EHR and claims data?
RWD sources are intended to serve specific purposes. None of those purposes are the conduct of validated, scientifically-sound clinical research into the benefits, risks, and efficacy of specific regulated medical products. The draft guidance re-states several of the discussion points about relevance of RWD, specifically for EHR and claims sources.
Claims data is used for billing, and may not “accurately reflect a particular disease,” especially if the patient does not use their insurance or the condition is not covered. For example, some patients may cash pay for certain drugs or services, such as certain contraceptive or reproductive health care or mental/behavioral or substance use treatment, which will then not be reflected in their claims data. In addition, claims and billing is unique to the coverage source. An individual that changes their coverage system (e.g., moves across state lines, changes jobs and therefore employer sponsored insurance, ages into Medicare or changes Medicare Advantage plans, churns in and out of Medicaid and the public exchange markets) will represent a “data break,” or a patient that is technically lost to follow up.
EHR data is typically used for clinical care, to generate billing information and to audit the quality of the clinical care provided (e.g., was a patient screened for common conditions before progressing to an invasive or expensive procedure). While the data captured in Certified EHR Technologies (CEHRTs) is standardized into a generalizable format, the data recorded within the EHR will depend on the practices of each specific site of service and their culture, practices, and system. Further, hospitals and larger practitioner offices must use CEHRT under Medicare payment policies, but some smaller or specific provider types (e.g., primary care, federally qualified health centers) may not – creating more data breaks. Similar to the limitations of claims data, EHRs will not capture information on care that is sought outside of the CEHRT system, such as vaccines received at work, experimental clinical trials, or certain sexual or behavioral health care.
Further, EHR data includes a unique challenge: that of unstructured data, typically found in free text fields. These data can be imperative to contextualize why a certain intervention was ordered or bypassed, including physician notes and PDF-based radiology reports. Although information technology (IT) and analytics have advanced in the capture and categorization of unstructured data, there is significant work to be done in order for these approaches to be feasibly relied upon for clinical research. For example, while AI methodologies (including natural language processing and deep learning) could link values and outcomes to unstructured data, “currently require a significant amount of human-aided curation and decision-making, injecting an additional level of data variability and quality considerations into the final study-specific dataset,” the FDA explains in the guidance. Any sponsor seeking to leverage such a methodology would need to clearly outline the model used, its operation and metrics of validation.
Notably, even data standardized into the ONC-accepted formats is still difficult to link, meaning that it is challenging to do a direct comparison of EHR records from one health care system to another, or to track a patient that may bounce between different systems.
Regulatory-grade RWD: Data quality, quality assurance, curation
Data from EHRs and claims information will have a “data lifecycle” in the transition from clinical and billing information into a regulatory-analyses-ready RWD analytic dataset intended for a specific study [for a visualization of the “lifecycle,” as FDA sees it, see page 25 of the draft guidance]. As such, the agency outlines “points for consideration” when assessing data quality at different points through its lifecycle.
At every stage of the data evaluation, the agency explains, sponsors and researchers should consider the “completeness, accuracy, and plausibility of the data.” Data plausibility, according to the agency, is “the believability or the truthfulness of data values.” Further, sponsors should assess data for “conformance” or “congruence with standardized types, sizes and formats.”
These practices should be outlined in detail in the protocol and analysis plan, including a description of how the data’s overall integrity (“completeness, consistency, and accuracy of data”) should be considered and the measures the developer took to bolster its integrity through evaluation and validation. Overall, the sponsor will need a robust quality assurance and curation plan, and a documented data management process.
The FDA outlines four key stages of the data lifecycle for the development and evaluation of a high quality RWD dataset. It recommends “automated data quality reports” that will assess the following key aspects of the lifecycle:
- Step one: Source data. The source data is the data as it exists in the real-world, including medical claims, birth or death certificates, and EHR data (e.g., provider notes, pathology/discharge/radiology/provider notes, demographics, diagnostic codes, lab results and visits). Data must be accrued from this source data, with a specific focus on the methods to retrieve the data that minimize missing data, ensuring the provenance (i.e., starting point) of the core data elements for tracking purposes, and timeliness. Accrual should also include processes for handling discrepancies and duplications in the records (e.g., the same subject represented twice, or differences in how different sites code). The sponsor should also consider the data’s capture – such as any coding changes or shifts in clinical practice that occurred during the study period or reason for (and timing of) any changes in processes by the personnel inputting the data.
- Step two: Curated data. The transition from source data to curated data typically requires manual curation – such as quality assurance and data quality tests across the disparate sites, documenting the clinical definitions and contextualizing the capture of core data elements, assessing data completeness and trends over time, and processing both the structured and unstructured data from its original source. Further, this is the phase in which data from different stages is harmonized into a common structure (which, “when applicable” should be based on existing, consensus-based data curation standards), and mapped accurate (e.g., from ICD-10 to SNOMED).
- Step three: Transformed data. The implementation of processes that extract, transform and load data into a warehouse. This may include, when necessary, de-identification of patient records. Data transformation also includes the standardization into consistent elements and semantics – or, if using a Common Data Model (CDM), the process by which the data would be transformed or translated into the CDM. This is the step in which a researcher would check the quality of record linkage and deduplication, quantify errors, and build procedures to adjudicate discrepancies.
- Step Four: Analytic dataset. The final, curated, transformed dataset should adhere to the specifications that were proactively laid out in the study protocol and the Statistical Analysis Plan (SAP). The sponsor will need to implement data checks to identify “implausible values” (e.g., weight, blood pressure) and the pattern of missing or implausible data. Researchers may conduct additional data transformations that are study-specific, such as those for specific subsets of patients.
The importance of data linking
The guidance also has recommendations regarding the use of distributed data networks and common data models (CDMs).
Distributed data networks are systems that incorporate data from a variety of other systems and organize the data. For health care purposes, these can include EHR, claim or registry data. Data networks can either be managed by a single entity responsible for its management and structure (the agency cites the Veterans Health Administration’s data network as an example) or leverage a hybrid model in which data from multiple partners is sent to a centralized repository (such as the CDC’s National Syndromic Surveillance system).
Further, a data network can be organized as a fully distributed system in which networks of data systems manage their own data (and structure if differently) but return answers to queries to a central location for reporting – such as the Sentinel Initiative. These networks can (and typically do) leverage CDMs to ensure that the data is managed and analyzed consistently. CDMs typically include “tools and methodologies for analysis, a consistent level of data curation, and periodic revision of the data model to incorporate new data concepts as needed.” In short, a CDM allows for different data-housing stakeholders to conduct the same inquiries, using the same methods, in comparably-designed and curated datasets, in a distributed way.
However, the guidance notes that distributed networks and CDMs can be challenging to work with, including concerns with missing data or insufficient relevant data elements with which to conduct specific queries. It recommends that sponsors review the data elements that are within the model (including whether they are “optional” elements that are unlikely to be included in a universal way across sites) before leveraging these methods. While analytics from a CDM can be supplemented with “customized study-specific data elements, collecting additional data, or using other data elements present in the dataset that are reasonable proxies for the missing information,” each of these new additions would need to be independently validated.
Facets of an RWD-based analysis
Identifying a research population:
As with all types of research, the “key variables used to select the study population should be validated.” However, there are some unique considerations for data from claims and EHR records.
The agency defines a computable phenotype of patient populations to be the selection based on methods that can identify similar patient populations from different sources. The definition of the phenotype, which includes data elements and the phenotype algorithm, should include metadata and supporting information about how it was defined, the intended use, the clinical rationale, research justification, and “data assessing validation in various health care settings.”
In identification processes, the agency recommends using specific codes or terms – such as the ICD-9 code for a specific health condition. It also acknowledges that some key variables may need to be leveraged from data entered by a provider (or triage personnel) at the point of care, such as the patient’s age. In all circumstances, the developer would need to describe the source of information and the methods used for capture (e.g., procedure to measure and enter blood pressure at the point of care).
Given the limitations in EHR/claims data in following a single patient over time comprehensively, the agency proposes that enrollment and disenrollment in different coverage systems “should be addressed” when leveraging both claims data and EHR sources – which the agency defines as continuity of coverage considerations. As the FDA explains, the data validity will depend on how, or whether, the data on a patient in “migrat[ed] into and out of health plans and health care delivery organizations.” Notably, this includes two main considerations for data sharing policy in the U.S.: interoperability for EHRs and data blocking provisions under HIPAA and the 21st Century Cures Act. While the Office of the National Coordinator for Health IT (ONC) has been working to advance interoperability through standardized data formatting and CEHRT requirements, the information blocking provisions are still very much a work in progress and something that would reasonably be outside of the control of a drug sponsor seeking to conduct clinical research.
Setting a time period and capturing data:
Given the potential for data breaks, the agency proposes that sponsors would be tasked with “specifying how all relevant populations, exposures, outcomes, and covariates” will be appropriately captured during the study period (i.e., the calendar time range of data used for the study). However, the agency does not propose a system or approach by which the data should be linked. Rather, the guidance indicates that sponsors would be responsible for identifying a specific methodology for capturing all data on the patients during the period of time specified for research.
While considerations of “missing data” are well-established for clinical researchers, RWD sources introduce a new consideration for data that is technically missing: data that “was not intended to be collected in the EHR and medical claims data and is therefore absent.” This varies from so-called traditional missing data in research, or when an element should have been captured but was not entered. For data that is missing in the non-traditional sense, the sponsor may potentially mitigate the impact on its study through data linking (discussed at length below) or by identifying a proxy-variable.
Defining your outcomes:
Operational versus Conceptual definitions: The concept of an “operational” definition was originally touched upon in the 2013 EHR data guidance. However, the idea of a “conceptual” definition is introduced in this new draft. In short, a “conceptual definition” of a health care condition is the clinical or scientific thinking about the variable, such as the clinical criteria for identifying an outcome or covariant. Operational definitions, then, are the specific methods and data capture needed to identify the conceptual definition. For example, if the conceptual definition of drug exposure is a measurement of drug intake, then the operational definition includes factors like the prescription order in the EHR, pharmacy codes, and/or a billing claim for filling the prescription.
However, assumptions of operational definitions may, themselves, lead to data validity concerns. In particular, misclassification, in which the operational definition may not classify the variable of interest for every subject or represented patient. For example, a patient may be using the drug of interest off-label, or may received a compounded prescription outside of a traditional pharmacy. A particular confounding factor, especially for drug research, is that pharmacy fill data does not always align with medication adherence. In general, sponsors would need to consider the variety of ways that their conceptual definitions could fail, the FDA recommends, including assumptions that they would and the magnitude that the resulting misclassifications would have on their study results. It also recommends pressure-testing the operational definition “in an adequately large sample of the population.”
A key best practice, the agency notes here, is to ensure that “complete verification” of the variables, which the agency defines as ensuring the accurate variable is assigned for each individual study subject “based on a reference standard” – a term the agency defines as “the best available benchmark” or “gold standard.” However, the guidance acknowledges that there are circumstances in which this is not feasible; for example, when there is no reference standard (i.e., established benchmark) that is available for all study subjects.
Measuring inputs and outputs
Exposure: Identification and Validation
The definition and description of an exposure to the treatment (i.e., product of interest) will need to include information about the drug dose (including any estimated cumulative dose), formulation, strength, route, timing, frequency, and (if relevant) duration. Further, “it may also be necessary” to identify specific manufacturers – for example, different vaccines.
The draft guidance outlines a series of considerations for how to ascertain a specific exposure, including the applicable or potential data sources, the duration of exposure and the dose. For example, researchers would need to identify what specific coding they are intending to use, and how granular those codes get. For example, an injectable drug’s NDC code would be recorded in a pharmacy, while a HCPCS J code would represent the same drug in a provider office. Further, the guidance acknowledges that “it is not always possible to infer” specific products from billing or diagnostic codes alone (e.g., multiple vaccines are coded under single billing codes), while some drugs may be completely unrepresented in claims (e.g., cash pay, discount programs, or samples). As previously mentioned, data has demonstrated that pharmacy fills are not necessary indicative of medication adherence – in effect, a patient may be represented in the pharmacy and billing data as compliant with a medication regimen, but may be diverting the drug, not taking it as prescribed or stockpiling it. A study protocol would need to identify “how researchers will measure use,” including the potential for early re-stocking (e.g., a patient loses a prescription) or concomitant medication (e.g., drugs dispensed on the same day, overlapping day supplies or two or more medications filled) and how that will impact dose or duration exposure.
The suitability of the data source will vary by the expected duration – while a vaccine is administered once, its effects are likely to be maintained for years, while an infusion drug may need monthly administrations and wear off more quickly – so sponsors should consider these data needs when considering specific data sources.
Outcomes: Identification and Validation
Similarly, “a crucial step in selecting a data source is determining whether it captures the clinical outcome of interest,” warns the draft guidance. The originally intended purposes of EHR and claims data tend to limit insight into mild symptoms that could be considered meaningful in clinical research, while “discrete outcomes or acute events” will be better documented than “worsening of existing problems” until that concern becomes a discrete event (e.g., gradually worsening pain becomes surgery). Without the ability to follow-up at pre-determined time points, the FDA raises concerns that EHR/claims data will not represent the monitoring necessary to assess outcomes, nor the standardization to group certain outcome measures together. In general, sponsors should discuss their proposed definitions of an outcome with the FDA well in advance of launching a study, and the agency outlines the need for a rigorous to assessing outcomes, including a quantitative bias analyses.
To align analyses with what is likely to be possible in RWD sources, the draft guidance recommends that the conceptual definitions need to be “able to be operationalized in RWD sources.” For example, studies related to “outcomes that are more subjective or scaled in nature,” such as worsening of pain or depression symptoms, may not be well suited to these analyses. Instead, conditions with “well-defined diagnostic criteria that are likely to be consistently captured in RWD, such as stroke, myocardial infarction or pulmonary embolism” may be “easier.”
Ascertaining the outcome, or applying the operational definition to the conceptual definition, sponsors should leverage the appropriate diagnostic and procedure codes, laboratory tests and values, and/or unstructured data.
The agency outlines a chart (page 12 of the draft guidance) to consider the “sensitivity” and “specificity” of an operational definition, or how functionally applicable and accurate the definition turns out to be (and the potential for misclassification). When ascertaining outcomes, the agency recommends that sponsors consider the positive predictive value (PPV) and negative predictive value (NPV) of the operational definition – or how likely subjects are to be misclassified. For diagnostic product, PPV and NPV are defined in context with prevalence, or how common the target is in a specific population. For RWD analyses, the guidance explains, there are some unique considerations, including “database-specific sensitivity,” or different patient demographics in different sites. However, the PPV and NPV – and the assessed degree of misclassification – will be critical to the validation of outcomes.
Overall, the agency recommends a rigorous validation process. While the validation should minimize outcome classification, it again acknowledges that “complete verification” may not be possible for all subjects. It recommends a standardized medical record review process, which should be reproducible, to minimize variability especially between sites. When complete verification is not possible, a “commonly used approach is to assess the performance of an operational definition in validation studies,” including assessments using the PPV and NPV. Further, performance should still be assessed, but using a “proposed study population using a justified sampling strategy.” Even in these circumstances, however, “misclassification remains a threat to the study internal validity, and the impact on the measure of association between exposure and outcome varies depending on whether the degree of misclassification differs between the exposure groups.”
Interestingly, the draft guidance specifically highlights the use of death as at outcome, noting that death is not typically encoded in an EHR (unless the patient dies while in inpatient care), nor is it a billing claim for reimbursement. The agency recommends that patients “who appear lost to follow-up” be run through searches of vital statistics.
Interestingly, there are some creative approaches that have been used for these purposes. For example, researchers from Flatiron have used EHR data in combination with end-of-treatment notes, condolence letters, obituaries, and Social Security Death Index (SSDI) data to try to validate a mortality endpoint for oncology care and research in RWD.
Covariates: Identification and Validation:
When considering covariates in RWD datasets, explains the draft guidance, there are two key elements: confounders and effect modifiers.
For now, the draft offers limited information on how the FDA will consider confounders in RWD analyses, noting that “epidemiologic and statistical methods for identifying and handling confounding in studies will be addressed in future guidance documents on RWE study design.” Instead, the agency notes that source data should be assessed for its ability to capture confounding data – for example, electronic health care data is unlikely to represent race/ethnicity, lifestyle factors or self-pay drug use (including illicit drug use) adequately. The guidance offers even more limited considerations for effect modifiers, or factors that may explain variability in treatment effects, simply noting that they should be “examined in the study, and relevant effect modifiers should be available in the chosen data source.” In general, the agency recommends that researchers validate, justify and describe the operational definitions of any confounders or effect modifiers to the best of their ability.
What’s Next
Comments on the draft guidance are due by November 29, 2021.
Overall, the draft guidance outlines the types of complexities a sponsor would need to consider when considering an RWD-based analysis. The guidance predominantly focuses on the quality of the baseline data and how to transition information in an EHR or a claims database into “regulatory-grade” RWD. However, the guidance provides limited concrete recommendations about how to avoid the myriad potential pitfalls it identifies, rather stating that sponsors will need to ensure their data’s quality and the validation of their selected measures and endpoints.
The guidance comes after the agency has recently focused on understanding and evaluating baseline RWD as a key barrier to effectively using RWE. For example, there has been increased focus from FDA’s leadership on baseline data and how to validate it for specific uses, especially following the lessons of the pandemic. Recently, the FDA’s Sentinel Initiative announced that it was taking on projects to assess the quality of two highly complex RWD sources (EHR data and Medicaid program data), even as the FDA has increasingly sought to leverage RWD for post-market uses. Notably, the operationalization of this draft guidance is likely to be informed by all of these research efforts.
This draft guidance itself is likely to be critical to the operation of the RWE Pilot program. As AgencyIQ has previously explained, under this pilot the FDA will review – and publicly discuss – the RWE analyses of volunteers. The approach is intended to bolster the agency’s own understanding and experience in reviewing RWD and RWE, by using a public forum and volunteer pilot cases to assess regulatory challenges and opportunities for RWE. The tests cases under the pilot are likely to be assessed according to the preliminary rubric laid out in this draft guidance, at least partially.
Interestingly, as mentioned above, the medical device industry has been able to leverage RWE in regulatory submissions for several years, leading to a more advanced regulatory framework for these regulated products. However, several of the challenges outlined by the new draft guidance also still plague the conduct of RWE analyses in the device industry – even after years of experience. Going forward, the life sciences industry may see similar challenges in the drug space, even following the recommendations in the guidance.
For example, the draft guidance outlines the complexities associated with identifying an appropriate operational definition, or clinical/scientific criteria, for a condition of interest. While the draft recommends some well-document conditions with well-established diagnostic criteria, recent experience indicates that this may still be a challenge. For example, an RWD-based analysis recently conducted in partnership with three health systems and the device firm Medtronic (and presented at a medical device RWE forum) ran into exactly the challenge outlined in the guidance document, even though the study sought to characterize an incredibly common and well-researched health condition: hypertension. However, the researchers ended up developing three “operational definitions” for controlled versus uncontrolled hypertension, but still highlighted concerns about the definitions’ robustness. At the meeting, CDRH’s Douglas Silverstein highlighted the lack of standardized definitions as a key limitation of the study design from a regulatory perspective.
Further, experience with devices demonstrates that situations in which a product is not adequately or uniquely identified in records can severely limit research. Medical device Unique Device Identifiers (UDIs) are not required to be entered into electronic health data capture at the point of care – while some providers have adopted such practices voluntarily (e.g., scanning at the point of care), UDIs are not required on claims forms. This has been cited as a key barrier to studying specific device products. As the field of RWE for drugs advances, this concern is likely to be present as well – especially for drugs for which a patient may be more likely to self-pay (based on insurance design, cash price or coupon availability) or codes covering multiple specific products (e.g., the vaccine codes).
However, it’s likely that drug-specific challenges will be uncovered as well, as stakeholders get more experience working to categorize, evaluate and analyze the underlying drug RWD. While some of these issues may be new for the FDA to grapple with, at least some are well-established in the U.S. health care system. For example, myriad stakeholders have raised concerns that EHR and claims data tend to be concentrated at the coasts, and especially in urban and higher-income areas, with limited representation of rural or underserved communities.
Further, data in EHRs and in claims include specific biases that reflect the incentive structures in which they were originally designed – baseline data factors that may confound analyses. In EHR data, this is demonstrated by the ongoing efforts around interoperability and prohibitions against data blocking. When federal incentives to use Certified EHR Technology (CEHRT) under the “Meaningful Use” program in 2011, there was no requirement for technology vendors that these systems be able to communicate with each other, leading to system-specific information siloes that CMS/ONC are still working to address.
From a claims perspective, financial incentives are often aligned with the data that is represented. For example, the Medicare program already routinely collects a significant amount of data routinely, which is structured in a uniform and standardized way. Theoretically, this makes the dataset ideal for RWD-based analysis – in fact, a recent review of RWE for regulatory use in medical devices cited the Centers for Medicare and Medicaid Services (CMS) database as an RWD source for 13 (out of 90) case studies, making it the more commonly used RWD source in the report. However, the Medicare data has specific quirks and biases that reflect the purpose it’s intended to serve that could impact regulatory research for life sciences companies.
For example, in the draft guidance the FDA proposes use of the Part D Low Income Subsidy (LIS) (i.e., financial assistance provided to certain beneficiaries in the prescription drug benefit) as a potential proxy measure for low socioeconomic status in an RWD dataset. However, the context of the LIS and how it operates may be a significant confounding factor in any sort of research analysis. Eligibility for the LIS is based on a set of resource limits, and can be either “full” or “partial” subsidies, which theoretically sets it as a good indicator for socioeconomic status. Currently, a little over a quarter (27%) of all Part D beneficiaries receive the LIS, and the share of LIS beneficiaries is increasing faster than the total Part D beneficiary cohort.
However, the Part D (prescription drug) benefit has a fundamentally different design for beneficiaries whether they are LIS or non-LIS – which impacts the way that beneficiaries seek and choose care. As the Medicare Payment Advisory Commission (MedPAC) puts it, “there are two distinct standard Part D benefit designs.” In context, Part DLIS beneficiaries are operationally insulated from prescription drug costs, paying only “nominal copayments set by law.” As such, LIS beneficiaries have demonstrated different behaviors than non-LIS beneficiaries, especially when it comes to selecting specific drugs (e.g., LIS beneficiaries are particularly less likely to choose generics, as the price difference between a higher-cost and lower-cost option for these patients is nonexistent or nominal). While this effect is well understood in Medicare payment data, it may skew research in Part D beneficiaries.
Similarly, the Medicare program faces challenges with so-called “coding intensity.” In short, there are demonstrable differences in the way that the same services are coded for under the traditional Medicare Fee-For-Service (FFS) program and the Medicare Advantage (MA) program. As MedPAC explains, “plans benefit from coding more comprehensively by gaining advantage through the determination of extra benefits.” In short, there is a financial incentive for claims in Medicare Advantage to be coded more intensely than those under FFS – which has led to variability in the way that MA and FFS data are represented in coding and claims information, with similar beneficiaries in MA represented by higher risk scores (i.e., they seem sicker) than those in FFS. While CMS is addressing the issue through risk adjustment, and the risk score disparities are closing, the issue of coding intensity may be relevant for clinical research.
While stakeholders like CMS, Congress and MedPAC track and research these issues due to overall cost concerns, it’s not yet clear how – or if – these various incentives will skew or be controlled in RWD analyses for regulated industry.
To contact the author of this item, please email Laura DiAngelo.
To contact the editor of this item, please email Alec Gaffney.
Key Documents and Dates
- FDA: Real-World Data: Assessing Electronic Health Records and Medical Claims Data To Support Regulatory Decision-Making for Drug and Biological Products
- Docket No. FDA-2020-D-2307
- Guidance Document Backup Download: 34193775fnl.pdf